Research
Research Direction
We work on distributed/federated machine learning, robust AI and resource-efficient AI, addressing all key issues in the deployment of practical AI systems
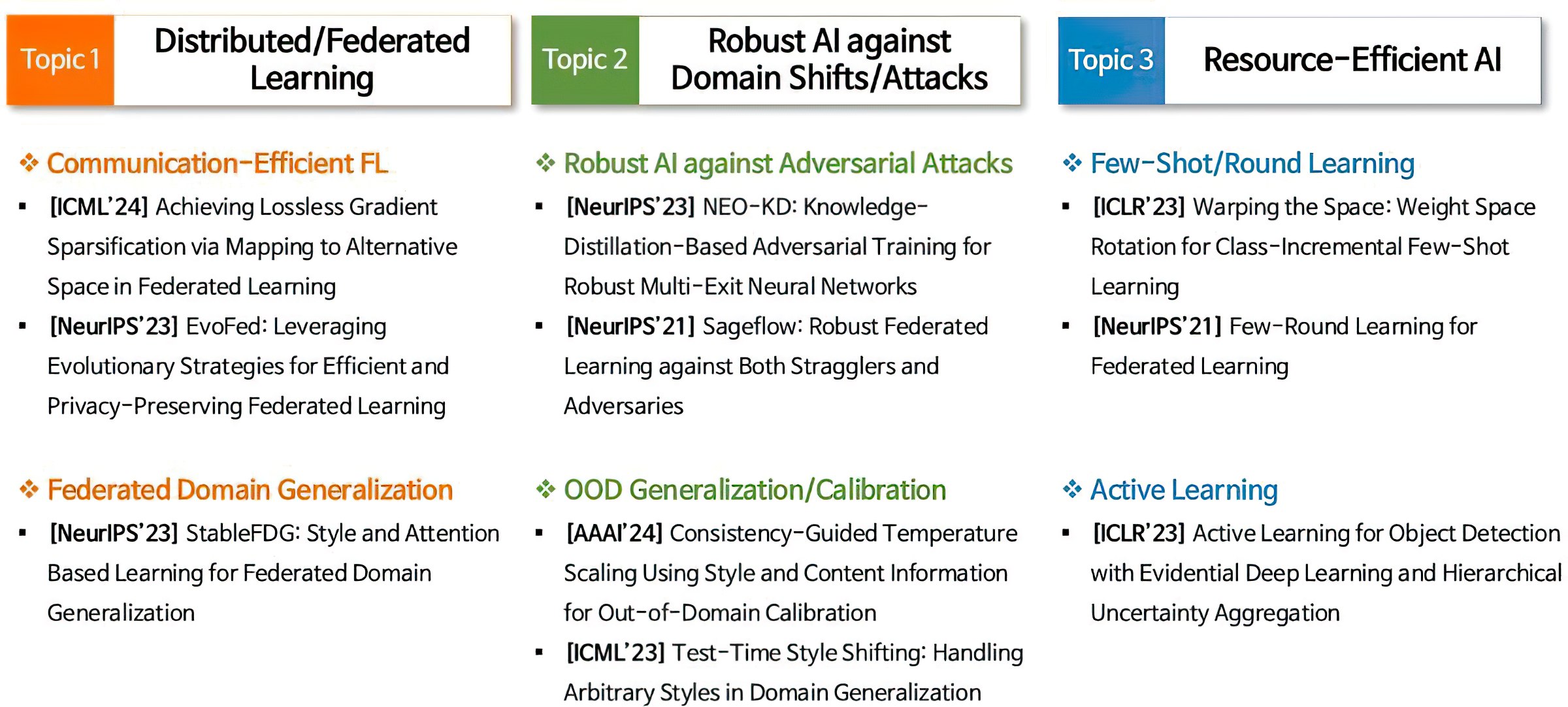
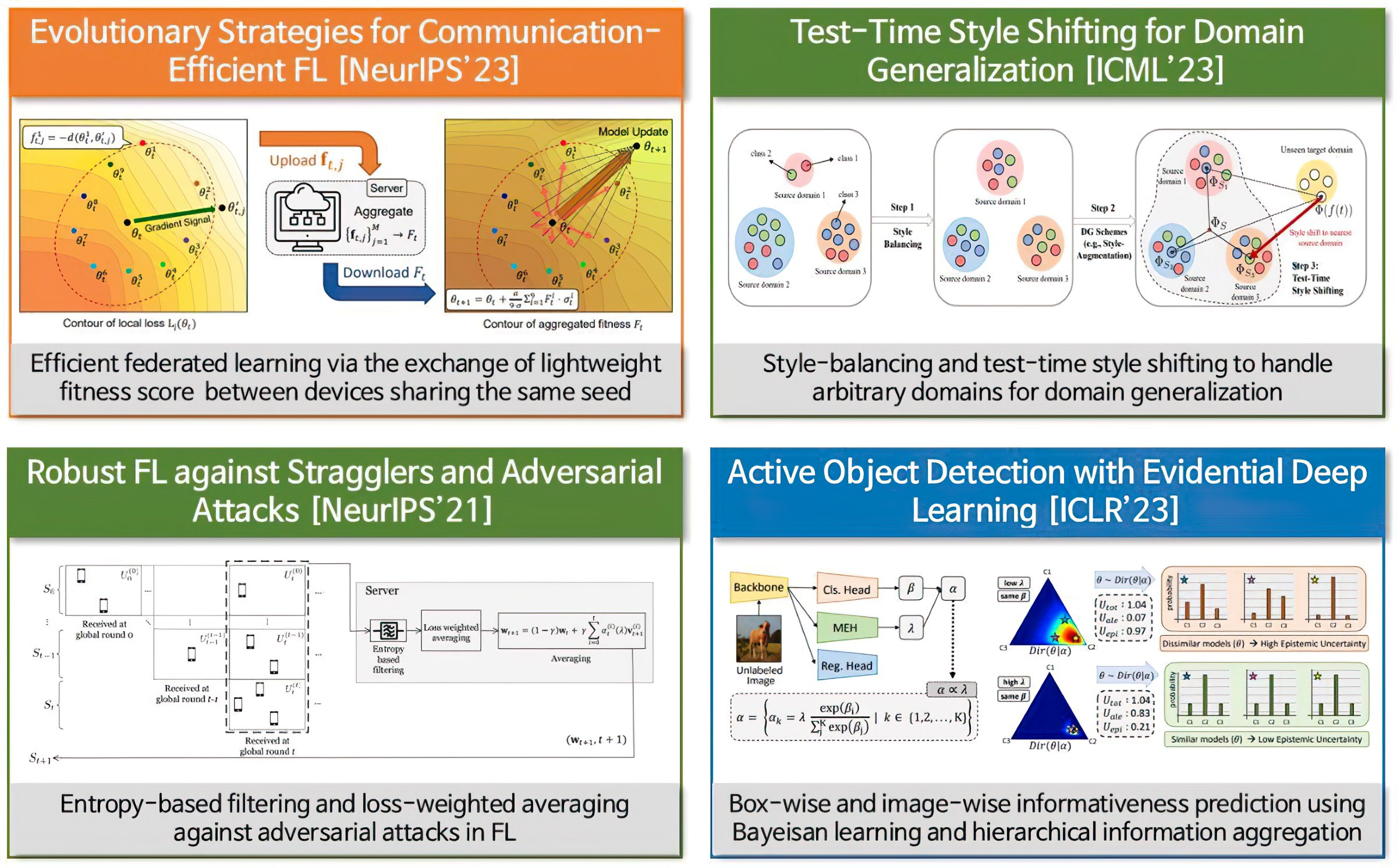
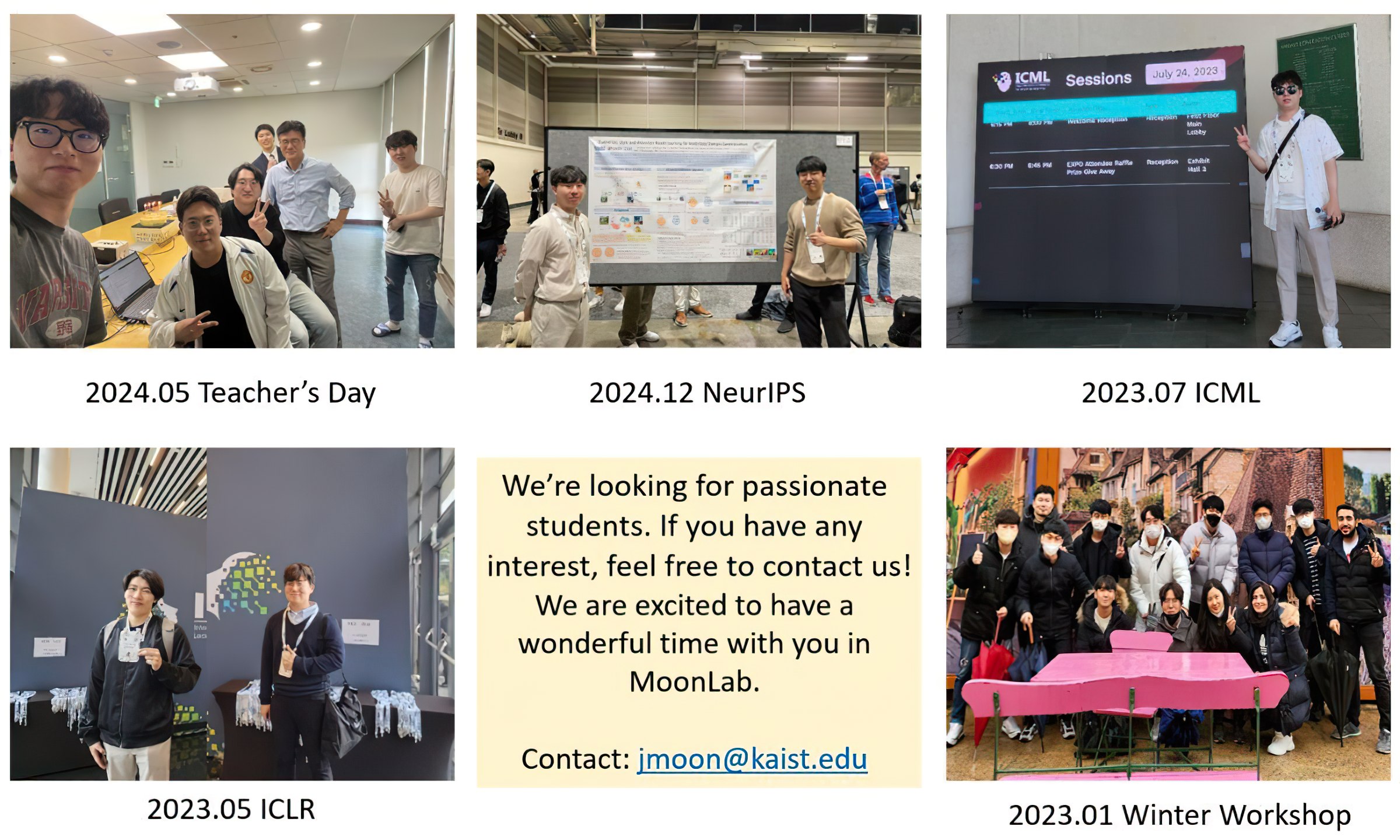
Research Direction
We work on distributed/federated machine learning, robust AI and resource-efficient AI, addressing all key issues in the deployment of practical AI systems
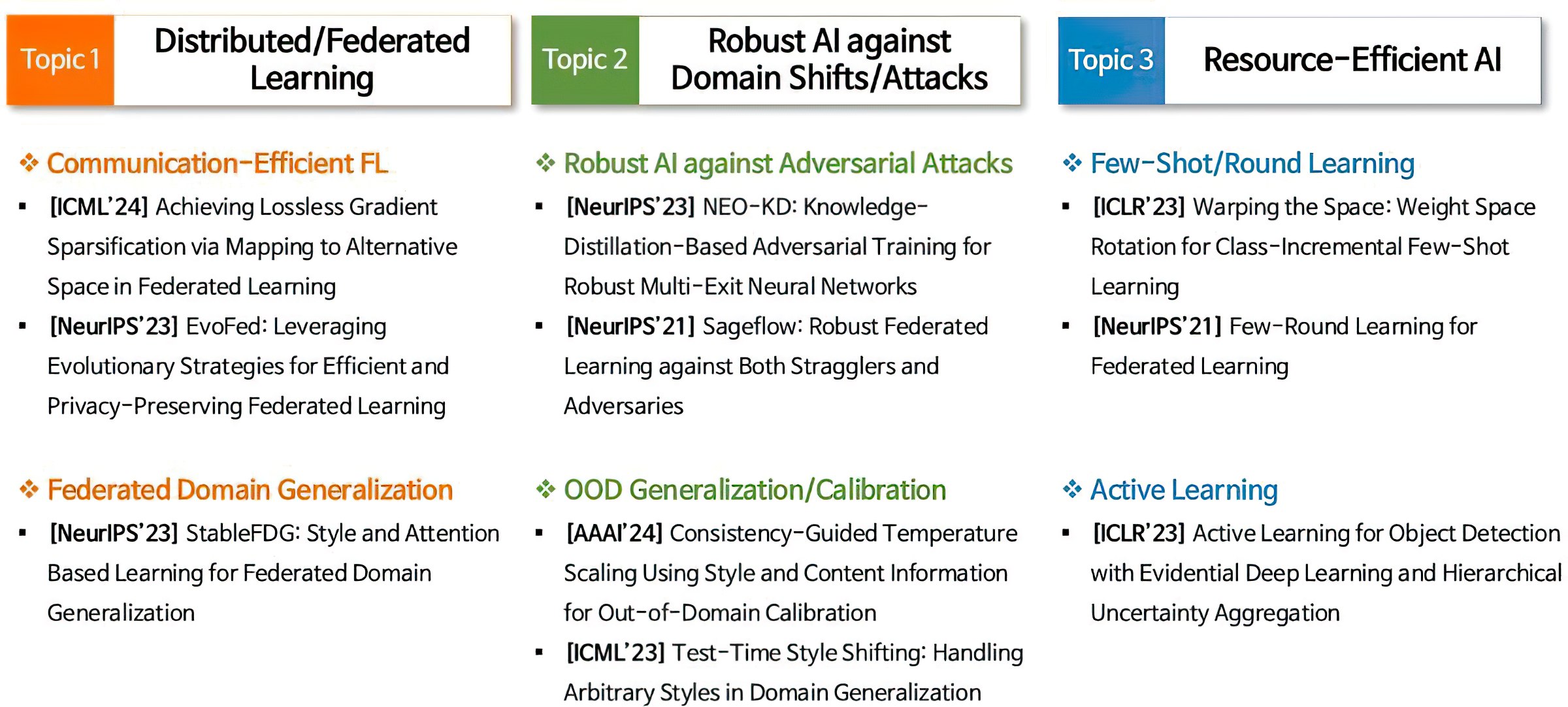
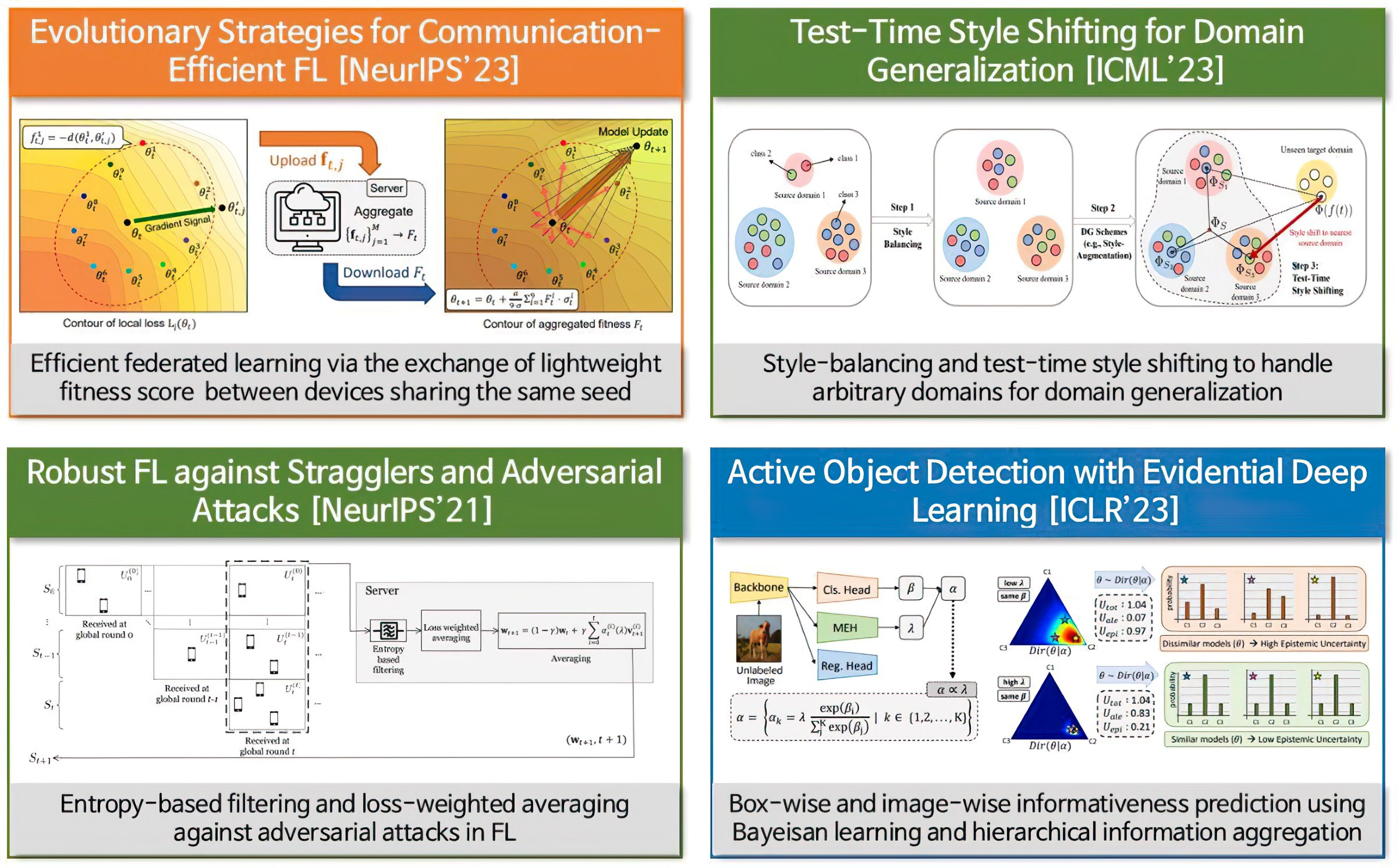
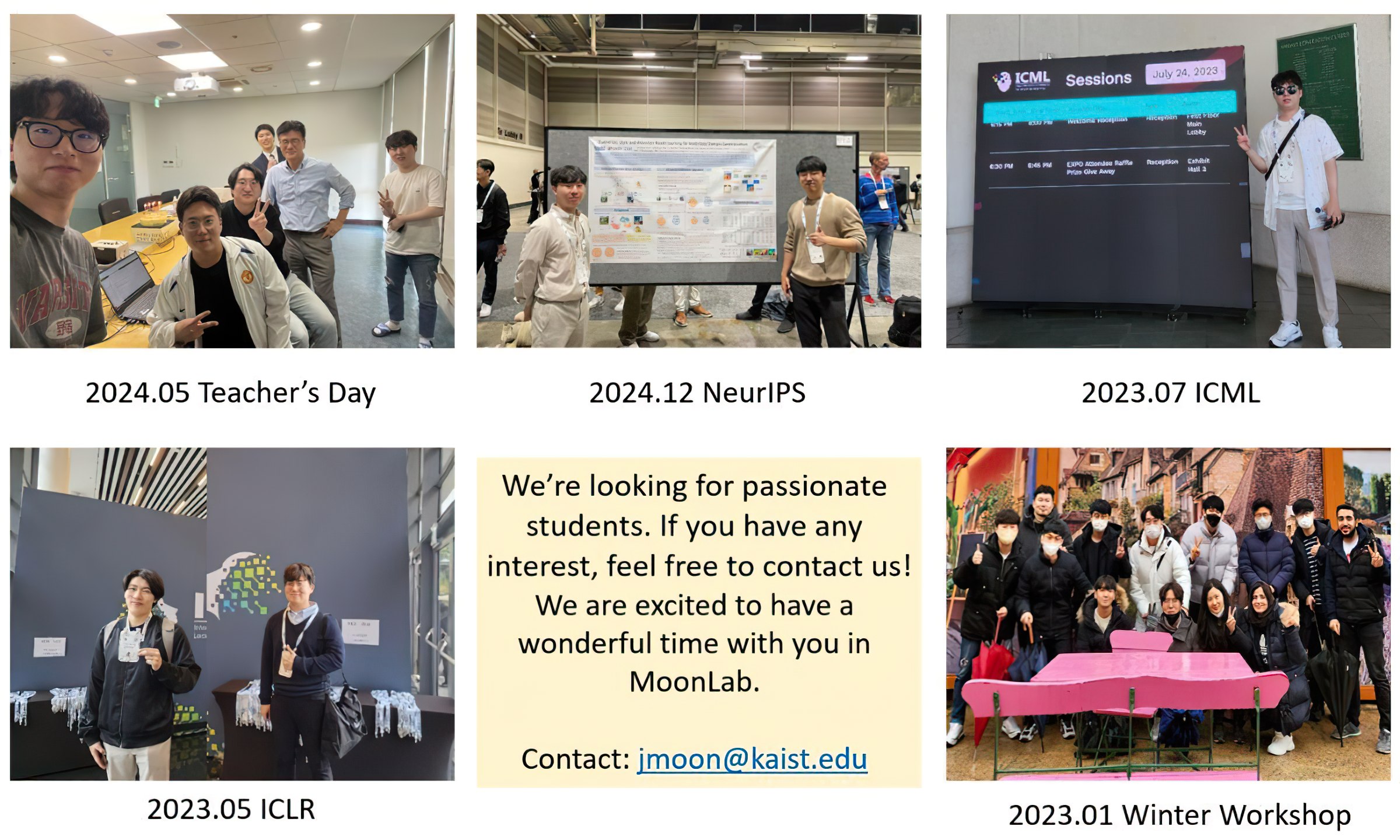